Application of Google Earth Engine for Monitoring Mangrove Forest Changes in Satun Province
Keywords:
Google Earth Engine , mangrove areas , random forest , remote sensing , Sentinel-2Abstract
Background and Objectives : Satun Province has rich and ecologically important mangrove forests that play a vital role in both the ecosystem and the economy. However, a continuous decline in these mangrove forests has been observed over recent years. Challenges within the study area include limited human resources, which impede effective monitoring and management. Furthermore, conventional remote sensing techniques necessitate specialized hardware, software, and data processing capabilities, thereby imposing limitations on accessibility and efficiency for certain organizations. This study aims to propose a methodology for utilizing Google Earth Engine (GEE), a powerful cloud-based platform for online satellite imagery analysis, to monitor changes in mangrove forest areas in Satun Province, covering the districts of Thung Wa, La Ngu, Tha Phae, and Mueang Satun. This study, covering the six-year period from 2018 to 2023, pursues several key objectives: to mitigate conflicts arising from human encroachment on mangrove ecosystems, to minimize hardware and software costs associated with traditional monitoring methods, and to address the critical issue of mangrove forest decline attributed to persistent encroachment and land use change.
Methodology : This study employed Sentinel-2 Multi Spectral Instrument (MSI) Level-1C and Level-2A imagery acquired during periods of minimal cloud cover over the study area within the specified year. Cloud masking procedures were implemented to mitigate cloud contamination, and vegetation indices, including the Normalized Difference Vegetation Index (NDVI), Soil Adjusted Vegetation Index (SAVI), and Enhanced Vegetation Index (EVI), were computed to generate training data for supervised land cover classification. Supervised classification was performed using the Random Forest algorithm, a robust machine learning classifier, with 30 iterations, implemented within the Earth Engine Code Editor on the Google Earth Engine (GEE) platform. Classification accuracy was assessed through field surveys and comparison with reference data derived from Google Earth. Furthermore, land use and land cover (LULC) change analysis was conducted for the period spanning 2018 to 2023 using Geographic Information Systems (GIS). Change detection analysis was performed by overlaying the annual classified datasets to identify areas of both persistence and change. The resulting land cover was categorized into six key classes relevant to the study area: natural water bodies (W1), mangrove forests (F3), aquaculture farms (A9), built-up areas (U2), agricultural areas (A0), and miscellaneous areas (M4). LULC changes were then summarized in a time series format to quantify and visualize the dynamics of land cover change.
Main Results : The integration of Google Earth Engine (GEE) with the Random Forest (RF) algorithm, a powerful machine learning classifier, significantly enhances the efficiency of mangrove forest change detection. Sentinel-2 satellite imagery, accessed through the Earth Engine Data Catalog, was processed using cloud computing resources, facilitating rapid and efficient analysis of extensive datasets. The land use and land cover classification achieved an overall accuracy exceeding 80% and a Kappa coefficient ranging from 0.6 to 0.8, based on 256 independent validation points strategically distributed across the study area. This validation, grounded in binomial probability theory, indicates an acceptable level of reliability for the classification results. The resulting data offer significant potential for effective mangrove resource management. The land use and land cover classification results showed the proportional distribution of land cover types in the study area. In 2018, mangrove forests constituted the largest proportion, covering 73.406% of the total area. This was followed by agricultural areas, which occupied 12.360%, natural water bodies at 1.803%, aquaculture areas at 7.278%, built-up areas at 0.873%, and finally, miscellaneous areas at 4.280%. By 2020, a notable shift in land cover was observed, with mangrove forest cover experiencing a decrease to 71.033%. This decrease was subsequently followed by a recovery, as mangrove forest cover increased to 72.300% by 2023. Analyzing the changes between 2018 and 2020 reveals a net loss of 2.373% in mangrove forest area. This loss was primarily attributed to the conversion of mangrove forest land into agricultural land, indicating a shift in land use practices within the study area. Conversely, the period from 2020 to 2023 witnessed a reversal of this trend, with mangrove forest area exhibiting a net increase of 1.267%. This increase can be primarily explained by the reconversion of agricultural land back into mangrove forests, suggesting a potential recovery or restoration of mangrove ecosystems.
Conclusions : The Google Earth Engine (GEE) platform facilitates the rapid online processing of satellite imagery, mitigating hardware limitations. However, the processing of very large datasets may necessitate segmentation into smaller tiles. For applications in other geographic regions, the definition of context-specific parameters and vegetation indices is crucial for achieving acceptable accuracy in land use and land cover classification. The findings of this study demonstrate the efficacy of GEE for monitoring changes in mangrove forest area over a six-year period. The observed expansion of mangrove forests between 2020 and 2023 can be attributed to the implementation of stricter governmental measures to prevent encroachment. This study represents a significant advancement in the application of satellite technology and geographic information systems (GIS) for mangrove forest conservation, offering a more cost-effective and time-efficient alternative to traditional field-based methods and enabling more efficient resource management. Furthermore, the developed methodology holds potential for future applications, such as estimating the carbon sequestration capacity of mangrove ecosystems.
References
Chen, B., Xiao, X., Li, X., Pan, L., Doughty, R., Ma, J., Dong, J., Qin, Y., Zhao, B., Wu, Z., Sun, R., Lan, G., Xie, G., Clinton, N.,& Giri. (2017) C. A mangrove forest map of China in 2015: Analysis of time series Landsat 7/8 and Sentinel-1A imagery in Google Earth Engine cloud computing platform, ISPRS Journal of Photogrammetry and Remote Sensing, 131, 104-120. doi.org/10.1016/j.isprsjprs.2017.07.011.
Department of Marine and Coastal Resources. (2018). Marine and Coastal Resources Data of Satun Province. Retrieved January 10, 2023, from https://www.dmcr.go.th/detailLib/3747.
Department of Marine and Coastal Resources. (2021). Assessment of Mangrove Forest Areas in Encroached Sites. Retrieved December 19, 2023, from https://www.dmcr.go.th/detailAll/52950/nws/22.
Huete, A., Didan, K., Miura, T., Rodriguez, E. P., Gao, X., & Ferreira, L. G. (2002). Overview of the radiometric and biophysical performance of the MODIS vegetation indices. Remote Sensing of Environment,83, (1–2), 195-213. doi.org/10.1016/S0034-4257(02)00096-2.
Huete, A. R. (1988). A soil-adjusted vegetation index (SAVI). Remote Sensing of Environment, 25 (3), 295-309 . doi.org/10.1016/0034-4257(88)90106-X.
Kingsley, K, & Bhuiyan A. (2024). Assessing changes in land cover, NDVI, and LST in the Sundarbans mangrove forest in Bangladesh and India: A GIS and remote sensing approach, Remote Sensing Applications: Society and Environment, 36, 101289. doi.org/10.1016/j.rsase.2024.101289.
Li, S., Zhu, Z., Deng, W., Zhu, Q., Xu, Z., Peng, B., Guo, F., Zhang, Y.,& Yang, Z. (2024). Estimation of aboveground biomass of different vegetation types in mangrove forests based on UAV remote sensing. Sustainable Horizons, 3(11), 100100 .doi.org/10.1016/j.horiz.2024.100100.
Lu, C., Liu, J., Jia, M., Liu, M., Man, W., Fu, W., Zhong, L., Lin, X., Su, Y.,& Gao, Y. (2018). Dynamic Analysis of Mangrove Forests Based on an Optimal Segmentation Scale Model and Multi-Seasonal Images in Quanzhou Bay, China. Remote Sensing, 10(12), 2020. doi.org/10.3390/rs10122020.
Mangrove Resources Promotion and Development Subdivision. (2016). Thai Mangrove Forest. Retrieved January 8, 2023, from https://dmcrth.dmcr.go.th/manpro.
Megha, B., Nophea, S., Takuji W., Manjunatha ,V., Issei .A,& Rajendra, P.(2024), Assessing changes in mangrove forest cover and carbon stocks in the Lower Mekong Region using Google Earth Engine, Innovation and Green Development, 3 (3), 100140. doi.org/10.1016/j.igd.2024.100140.
Nasiri, V., Deljouei, A., Moradi, F., Sadeghi, S.M.M., & Borz, S.A. (2022), Land Use and Land Cover Mapping Using Sentinel-2, Landsat-8 Satellite Images, and Google Earth Engine: A Comparison of Two Composition Methods. Remote Sens, 14 (9), 1977. doi.org/10.3390/rs14091977.
Pimple, U., Simonetti, D., Sitthi, A., Pungkul, S., Leadprathom, K., Skupek, H., Som-ard, J., Gond, V., & Towprayoon, S. (2017). Google Earth Engine Based Three Decadal Landsat Imagery Analysis for Mapping of Mangrove Forests and Its Surroundings in the Trat Province of Thailand. Journal of Computer and Communications, 6, 247-264. doi.org/10.4236/jcc.2018.61025.
Rouse, J.W., Haas, R.H., Schell, J.A.,& Deering, D.W., (1973). Monitoring vegetation systems in the great plains with ERTS. In: Proceedings of 3rd Earth Resources Technology Satellite-1 Symposium, Greenbelt, NASA SP-351, pp. 3010–3017.
Shelestov, A., Lavreniuk, M., Kussul, N., Novikov, A.,& Skakun, S. (2017). Exploring Google Earth Engine Platform for Big Data Processing: Classification of Multi-Temporal Satellite Imagery for Crop Mapping. Sec. Environmental Informatics and Remote Sensing, 5 – 2017. doi.org/10.3389/feart.2017.00017.
Winarso, G., Rosid, M., Kamal, M., Asriningrum, W., Margules, C.,& Supriatna, J. (2023). Comparison of Mangrove Index (MI) and Normalized Difference Vegetation Index (NDVI) for the detection of degraded mangroves in Alas Purwo Banyuwangi and Segara Anakan Cilacap, Indonesia. Ecological Engineering, 197, 107119. doi.org/10.1016/j.ecoleng.2023.107119.
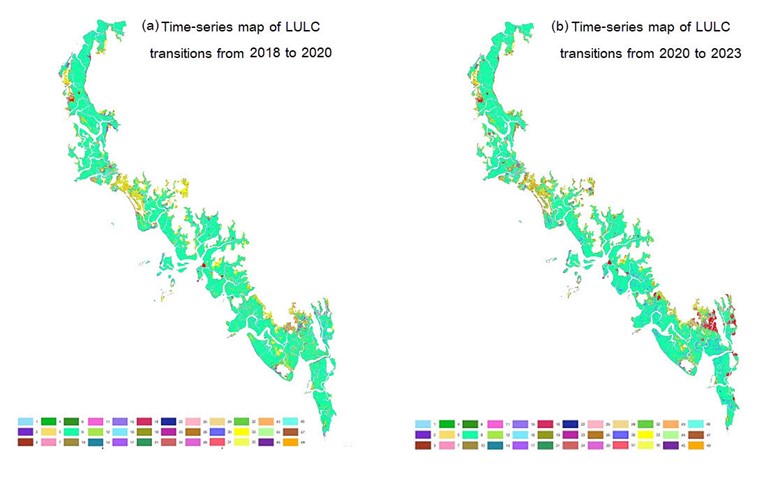
Downloads
Published
How to Cite
Issue
Section
License
Copyright (c) 2025 Faculty of Science, Burapha University

This work is licensed under a Creative Commons Attribution-NonCommercial-NoDerivatives 4.0 International License.
Burapha Science Journal is licensed under a Creative Commons Attribution-NonCommercial-NoDerivatives 4.0 International (CC BY-NC-ND 4.0) licence, unless otherwise stated. Please read our Policies page for more information