Analysis of Related Air Pollutants and Model for Estimating PM2.5 Concentration Using Satellite Images in The Upper Northern of Thailand
Keywords:
aerosol optical depth , PM2.5 , air pollutant , satellite image , modelAbstract
Background and Objectives : Air pollution is a condition in which the air is contaminated with pollutants or contaminants in large quantities over a prolonged period, posing a threat to living organisms. These contaminants can exist in solid, liquid, or gaseous forms. Various air pollutants adversely affect human health, including fine particulate matter (PM), carbon monoxide (CO), nitrogen dioxide (NO2), sulfur dioxide (SO2), lead (Pb), ozone (O3), and volatile organic compounds (VOCs). Being in an environment with air pollution increases the risk of developing serious diseases, such as respiratory diseases and cardiovascular diseases. Ground-based air quality monitoring in Thailand still faces several limitations, such as a limited number of monitoring stations and insufficient coverage in many areas, particularly in remote or rural regions where no stations are installed. This lack of coverage may negatively impact vulnerable populations in terms of health protection. Due to the nature of fine particulate matter, smoke, and air pollutants, these particles have the ability to absorb and scatter solar radiation, allowing only a portion of the radiation to reach the Earth's surface. As a result, aerosol optical depth (AOD) can be applied to assess the amount of solar radiation affected by airborne particles before reaching the Earth. AOD represents the relationship between the proportion of particles measured vertically above the ground and the concentration of particles at the surface level. This study aims to analyze the relationship between PM2.5 concentrations and related air pollutants in the upper northern region of Thailand and to develop a model for estimating air pollutant concentrations using daily satellite imagery from January 2014 to December 2023, totaling 2,721 images. The study compares results across different seasons to enhance the model’s efficiency and accuracy. The application of remote sensing technology not only improves air quality monitoring coverage but also supports policy planning, research, and long-term environmental management. This approach enables more effective responses to air pollution issues, contributing to better air quality management strategies.
Methodology : Data used in this study were; 1) Aerosol Optical Depth (AOD) data from the MCD19A2v061 product, obtained from the MODIS sensor onboard the Terra and Aqua satellites, which is used to analyze atmospheric aerosol levels; and 2) Ground-based measurements of PM2.5 and associated air pollutants, including carbon monoxide (CO), nitrogen dioxide (NO2), and sulfur dioxide (SO2), sourced from the Air Quality and Noise Management Bureau, Pollution Control Department. The relationship between PM2.5 concentrations and related air pollutants was examined using Pearson correlation analysis. Additionally, six regression models, including linear, logarithmic, quadratic, cubic, power, and exponential, were applied to determine the best-fit equation by incorporating AOD data with ground-based measurements of PM2.5 and air pollutants. After that, model validation was conducted by comparing the estimated concentrations of PM2.5 and air pollutants with the observed concentrations from ground-based monitoring stations.
Main Results : The study found seasonal variations in the correlation between PM2.5 and air pollutants. PM2.5 and CO had the highest correlation in summer (R2 = 0.789), moderate correlation in winter (R2 = 0.560), and the lowest correlation in the rainy season (R2 = 0.124). The correlation between PM2.5 and NO2, as well as SO2, was moderate in winter (R2 = 0.501 and R2 = 0.395, respectively), while the lowest correlations were observed in summer and the rainy season (R2 = 0.103, R2 = 0.018 for NO2 ; R2 = 0.002, R2 = 0.001 for SO2, respectively). The model results indicated that PM2.5 and CO ground-based measurements exhibited a cubic relationship with AOD data.
The summer season model demonstrated the highest accuracy, with R2 values of 0.923 for PM2.5 and 0.823 for CO. The model’s estimation of PM2.5 concentration achieved an overall accuracy of 92.5%, while CO concentration estimation reached 80% accuracy. A comparison of the monthly concentration trends between the model estimates and ground station observations showed an agreement between the two datasets.
Conclusions : The study highlights the seasonal variation in the correlation between PM2.5 and air pollutants, with the highest correlation between PM2.5 and CO occurring in summer due to biomass burning and forest fires, which are major sources of air pollution in northern Thailand. A moderate correlation was observed in winter, while the lowest correlation was found in the rainy season. The relationship between PM2.5 and NO2, as well as SO2, was moderate in winter due to the influence of temperature inversion, which traps pollutants. However, in summer and the rainy season, the correlations were very low, especially during the rainy season, when precipitation helps reduce pollutant concentrations through wet deposition. Northern Thailand frequently experiences severe air pollution, particularly with PM2.5 levels exceeding air quality standards from February to May. During this period, air pollution reaches levels that pose health risks, potentially causing both short-term and long-term health effects. This study developed a model to estimate PM2.5 concentrations and associated air pollutants using aerosol optical depth (AOD) data combined with ground-based air quality monitoring data. The findings indicate that the model, when applied to satellite imagery, achieves high accuracy and can be used in areas lacking ground-based air quality monitoring stations. This provides valuable support for relevant agencies in implementing more effective air pollution prevention and mitigation measures.
References
Asadi, A., Goharnejad, H., & Niri, M. Z. (2019). Regression modelling of air quality based on meteorological parameters and satellite data. Journal of Elementology, 24(1).
Bao, V. Q., & Van, T. T. (2021). An empirical relationship between PM2. 5 and aerosol optical depth from MODIS satellite images for spatial simulation over Ho Chi Minh city. Vietnam Journal of Science, Technology and Engineering, 63(4), 72-78.
Buya, S., Usanavasin, S., Gokon, H., & Karnjana, J. (2023). An Estimation of daily PM2. 5 Concentration in Thailand using satellite Data at 1-kilometer resolution. Sustainability, 15(13), 10024.
Chairattanawan, K., & Patthirasinsiri, N. (2020). Emission source impact and problem solving and management on PM2. 5 in the Northern part of Thailand. J Assoc Res, 25(1), 432-446.
Charoenpanyanet, A., & Hemwan, P. (2019). Suitable Model for Estimation of PM2. 5 Concentration Using Aerosol Optical Thickness (AOT) and Ground based Station: Under the Dome in Upper Northern, Thailand.International Journal of Geoinformatics, 15(3).
Kawichai, S., Prapamontol, T., Cao, F., Song, W., & Zhang, Y. L. (2024). Characteristics of Carbonaceous Species of PM2. 5 in Chiang Mai City, Thailand. Aerosol and Air Quality Research, 24(4), 230269.
Lalchandani, V., Srivastava, D., Dave, J., Mishra, S., Tripathi, N., Shukla, A. K., Sahu, R., Thamban, N. M., Gaddamidi, S., Dixit, K., Ganguly, D., Tiwari, S., Srivastava, A. K., Sahu, L., Rastogi, N., Gargava, P., & Tripathi, S. N. (2022). Effect of biomass burning on PM2. 5 composition and secondary aerosol formation during post‐monsoon and winter haze episodes in Delhi. Journal of Geophysical Research: Atmospheres, 127(1), e2021JD035232.
Niedźwiedź, T., Łupikasza, E. B., Małarzewski, Ł., & Budzik, T. (2021). Surface-based nocturnal air temperature inversions in southern Poland and their influence on PM10 and PM2.5 concentrations in Upper Silesia. Theoretical and Applied Climatology, 146(3), 897-919.
Onivefu, A. P., & Imarhiagbe, O. (2024). Types of Air Pollutants. Air Pollutants in the Context of One Health: Fundamentals, Sources, and Impacts (pp. 123-160). Cham: Springer Nature Switzerland.
Peng-In, B., Sanitluea, P., Monjatturat, P., Boonkerd, P., & Phosri, A. (2022). Estimating ground-level PM2. 5 over Bangkok Metropolitan Region in Thailand using aerosol optical depth retrieved by MODIS. Air Quality, Atmosphere & Health, 15(11), 2091-2102.
Satheesh, S. K., & Moorthy, K. K. (2005). Radiative effects of natural aerosols: A review. Atmospheric Environment, 39(11), 2089-2110.
Shahriyari, H. A., Nikmanesh, Y., Jalali, S., Tahery, N., Zhiani Fard, A., Hatamzadeh, N., Zarea, K., Cheraghi, M., & Mohammadi, M. J. (2022). Air pollution and human health risks: mechanisms and clinical Manifestations of cardiovascular and respiratory diseases. Toxin Reviews, 41(2), 606-617.
Shao, M., Xu, X., Lu, Y., & Dai, Q. (2023). Spatio-temporally differentiated impacts of temperature inversion on surface PM2. 5 in eastern China. Science of the Total Environment, 855, 158785.
Srianan, K., & Lalitaporn, P. (2022). Estimating particulate matter concentration by using satellite data over central Thailand. Journal of Environmental and Sustainable Management, 18(2), 4-25.
Tao, J., Zhang, L., Engling, G., Zhang, R., Yang, Y., Cao, J., Zhu, C., Wang, Q., & Luo, L. (2013). Chemical composition of PM2. 5 in an urban environment in Chengdu, China: Importance of springtime dust storms and biomass burning. Atmospheric Research, 122, 270-283.
Zhang, Y., & Li, Z. (2015). Remote sensing of atmospheric fine particulate matter (PM2. 5) mass concentration near the ground from satellite observation. Remote Sensing of Environment, 160, 252-262.
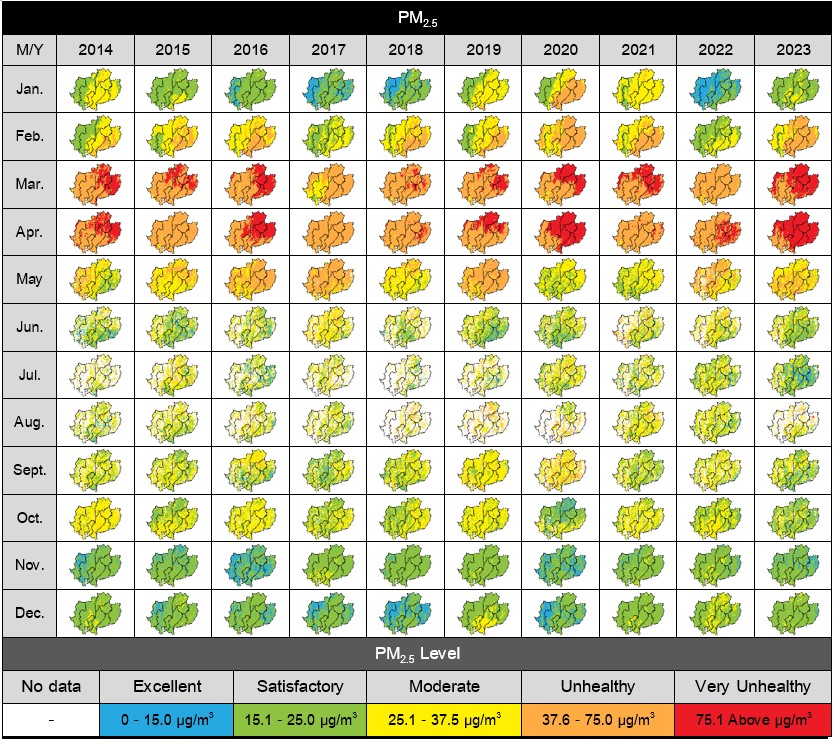
Downloads
Published
How to Cite
Issue
Section
License
Copyright (c) 2025 Faculty of Science, Burapha University

This work is licensed under a Creative Commons Attribution-NonCommercial-NoDerivatives 4.0 International License.
Burapha Science Journal is licensed under a Creative Commons Attribution-NonCommercial-NoDerivatives 4.0 International (CC BY-NC-ND 4.0) licence, unless otherwise stated. Please read our Policies page for more information